Corralling Machine Learning for LCRR Inventory Development
Learn about applying machine learning and predictive modeling to develop service line material inventories in our Corralling Machine Learning for Inventory Development webinar. CDM Smith’s LCR Compliance Coordinator Joanna Cummings, along with Trinnex’s leadCAST™ product manager Mark Zito, provide essential guidance in defining the rules of the road for machine learning.
Machine learning and other verification methods
When developing a service line inventory, there isn’t a 100% foolproof verification method to identify pipe materials, yet many of these methods are accepted by the EPA and most states. At an achievable accuracy rate of 80% or higher, machine learning, or predictive modeling, can be as or more accurate than other verification methods when reined in and used correctly. Developing machine learning and using it effectively can yield tremendous cost benefits to water utilities working on their inventories.
Machine learning is used to predict if a service line is highly likely to be lead based on historical data and other defined characteristics assumed to identify lead material, such as year of installation on the utility side, home construction date beyond a certain year, pipe diameter of a certain size, or other neighborhood and economic characteristics. It's used to correlate these characteristics with known materials from either targeted or random inspections. This process is then repeated to increase accuracy of the model.
Machine learning is an iterative process and is best suited for use alongside ongoing field verifications. There are two primary uses for machine learning. The first is planning and prioritization, and this can be done in the initial stages of the iterative process. The initial model is developed from any available material verifications, which can come from previous field work such as water main or meter replacement programs. The initial model can support utilities in prioritizing field inspections, estimating replacement costs, sanity checking confidence in historical records.
After the initial model is built, it needs to be periodically updated with new data, as more field verifications are completed. This is when the model’s hit rate and accuracy should improve. Towards the end of the iterative process, presuming the model has been enriched with new field verifications over time and its accuracy is comparable to other verification methods, we can consider using it to determine service line material in the inventory. It’s important to note that the EPA cites machine learning as a method to assign likelihood of lead to unknown services, but ultimately leaves the acceptance of machine learning as a verification method up to individual states.
Guidance from the EPA
To be in compliance with the LCRR, utilities must submit their materials inventory recorded for both the utility and private side of the service laterals by October 2024. Every single service line connected to the system, for potable and non-potable water use, must be included with annual updates on replacements and new information. Homeowners are notified if they are connected to confirmed lead service lines (LSL) or “lead status unknown” pipes, and inventories must be publicly available to meet the requirements of the LCRR.
The EPA released additional service line inventory guidance in August 2022 to advise how inventories should be developed and what information water utilities should use. Inventories are not a one-and-done action. Developing a utility’s inventory is a continuous process that becomes more accurate as more information is discovered. The more informed an inventory is, the greater level of certainty a utility can have when taking stock of their possible LSLs.
Under the federal rule, historical records are required to be reviewed in when developing an LSL materials inventory. Field investigations should then be selected based on the remaining unknowns. Any material information on hard copy and electronic records, such as tap cards or distribution maps, previous material evaluations, permit applications, and inspections must be included. In addition, historic construction and plumbing codes must be evaluated for relevance to water service line materials. Many states include additional types of reviews, so be sure to check local state requirements.
Leveraging machine learning
Machine learning is a subset of artificial intelligence (AI) that focuses on developing models through learned patterns in data. The model doesn’t rely on programming; it relies on using training data to identify patterns, automate them, and repeat them to identify possible LSL locations and their probability of containing lead. Machine learning can improve upon historical data, expand the impact of field verification methods, estimate where lead service lines are located, and decrease costs with targeted field verifications.
Machine learning models, like Trinnex’s leadCAST™ Predict, can be trained and tested by removing roughly 20% the services with known material (the “test set”) from the model's view, then training the model with the remaining 80% of services with known material (the “training set”) to predict the materials of the other 20% (the “test set”). By comparing predicted material to actual material for the 20% of records in the “test set,” we can assess how the model is performing. The initial results of the model test run can be used to identify targeted field verifications. The findings from these targeted field verifications are then fed back into the model through multiple iterations. As a result, the accuracy of the model should improve over time.
Keep in mind, machine learning models must be trained for each utility- they are not universal. Utility A may have banned lead in 1950, whereas Utility B enacted the federal ban in 1988. Additionally, if there is no lead in a training/test data set, traditional two-class classification models will not be able to determine features related to lead and make accurate predictions for lead services. Utilities can overcome this by training on the second-worst material in the service lateral, such as galvanized. Training the model with specific housing data in the area, instead of general census data, which is aggregated and not house-specific, will also yield more accurate results.
How can utilities get started?
Developing an inventory and initial steps to using machine learning are similar, so utilities do not need to fully commit to machine learning to get started.
Inputting third-party data sets into the model is helpful to train it. Parcel and tax assessor data, such as building age, lot size, home value, zoning and land use can inform the model of the possible presence of lead. Housing data such as age, square footage, number of beds and baths, and sale price are also key data sources that help the model learn. Demographic data like census tract or block and age of population are helpful for model training as well. If you need assistance selecting the proper input data for your machine learning model, contact Trinnex. Their dedicated team specializes in using predictive modeling to combat unknown service line materials using the leadCAST Predict™ model.
Machine learning is not an entirely new process—it is an option to automate a utility’s efforts to find lead more efficiently, informed by historical records and physical verifications. It helps fill in the gaps to make records as useful as possible, improves accuracy, and reduces expensive, disruptive, and time-consuming field verifications. By combining machine learning with human intelligence, communities can improve their service line inventories and focus efforts on improving public health in a more efficient and cost-effective way.
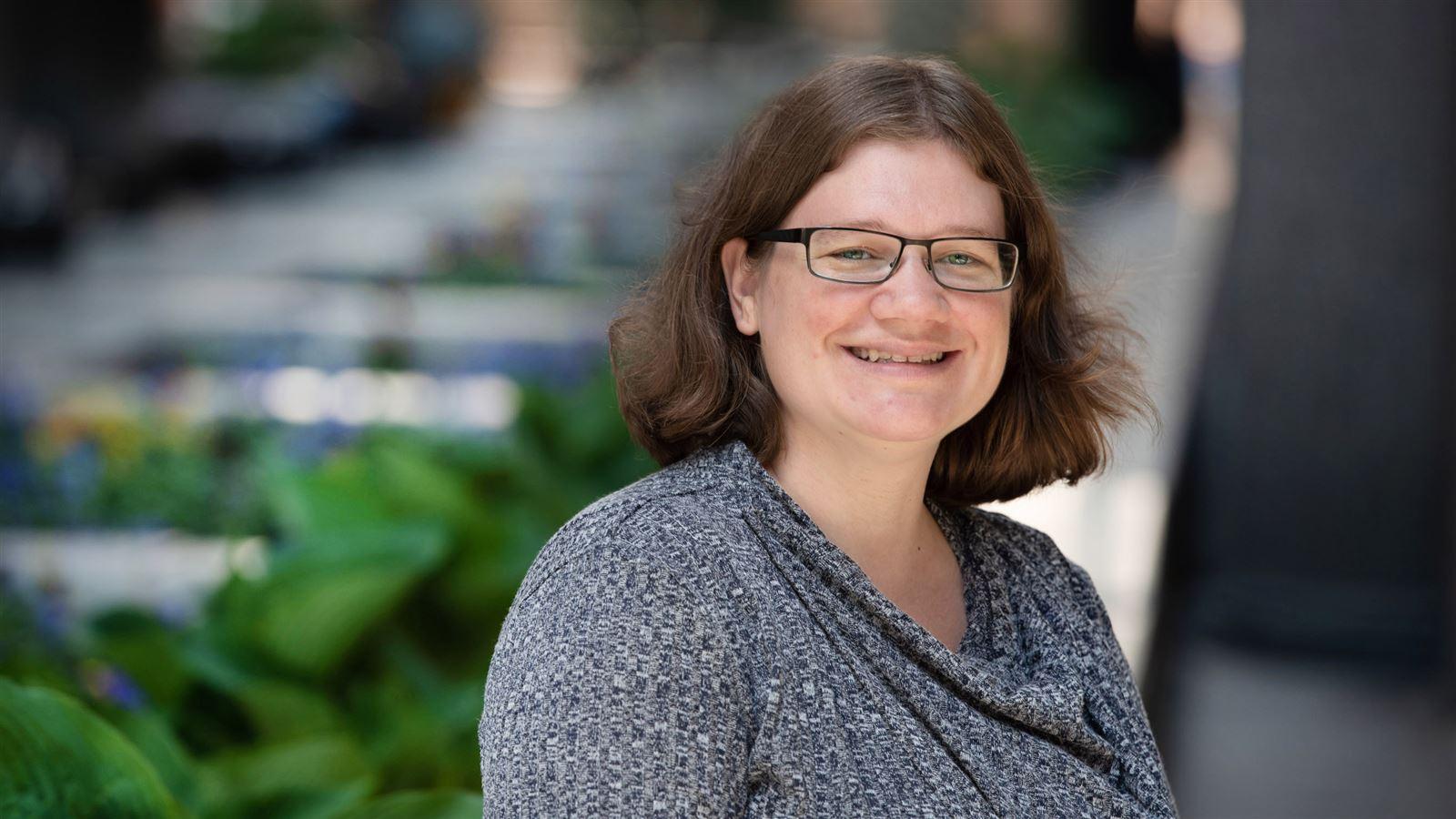
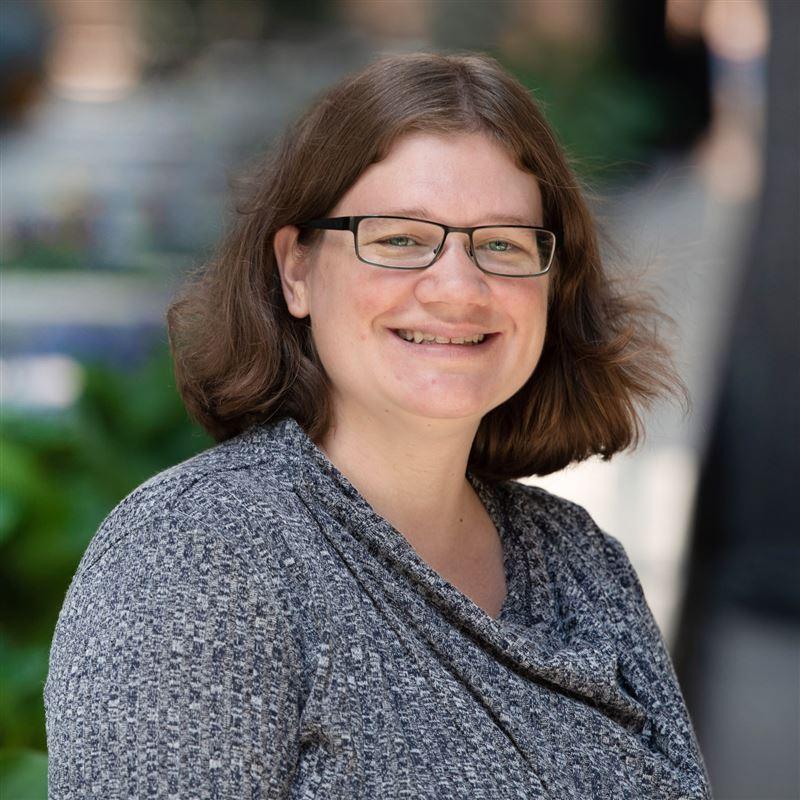
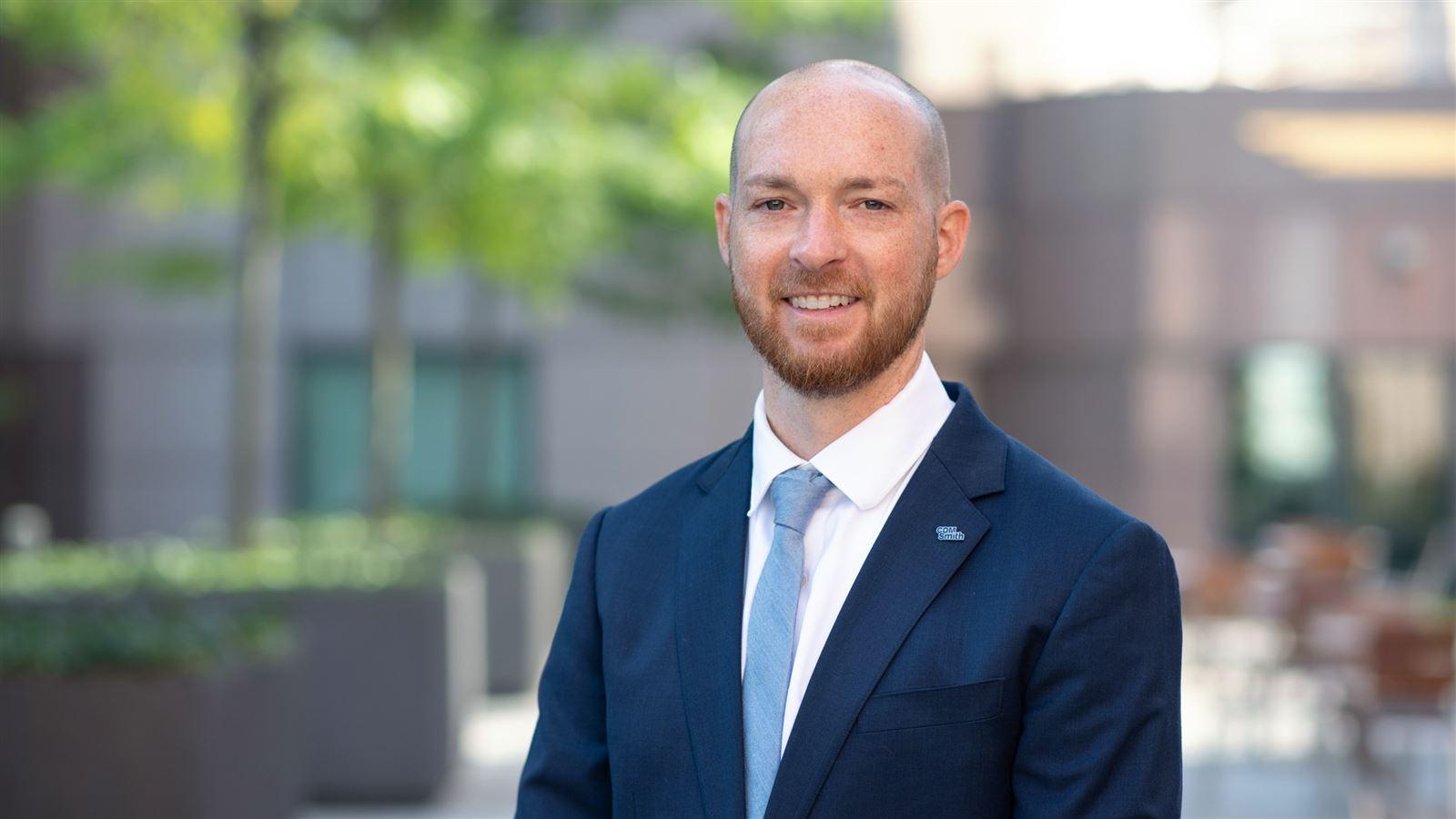
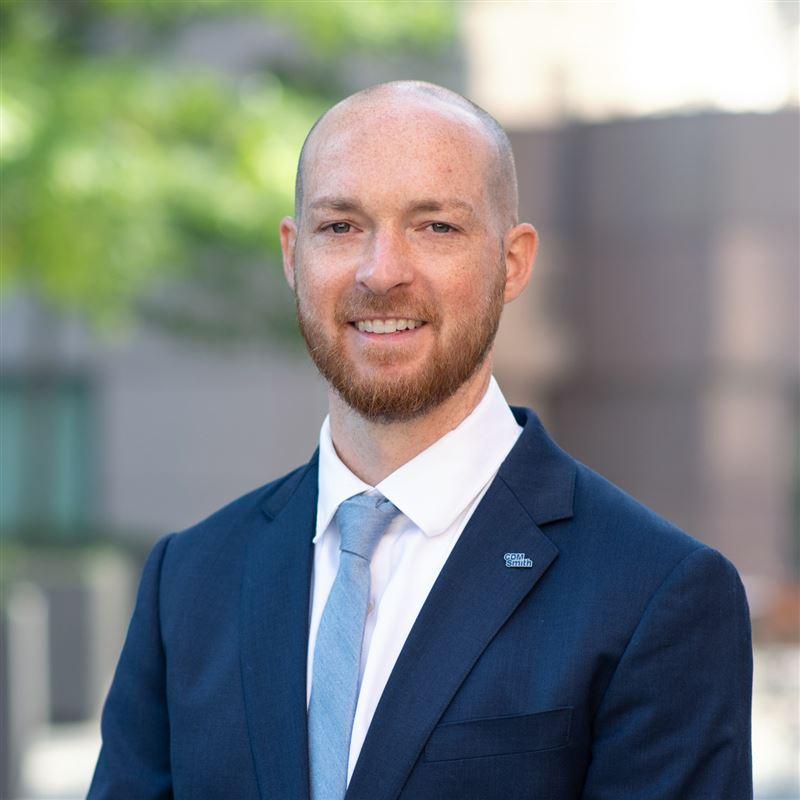
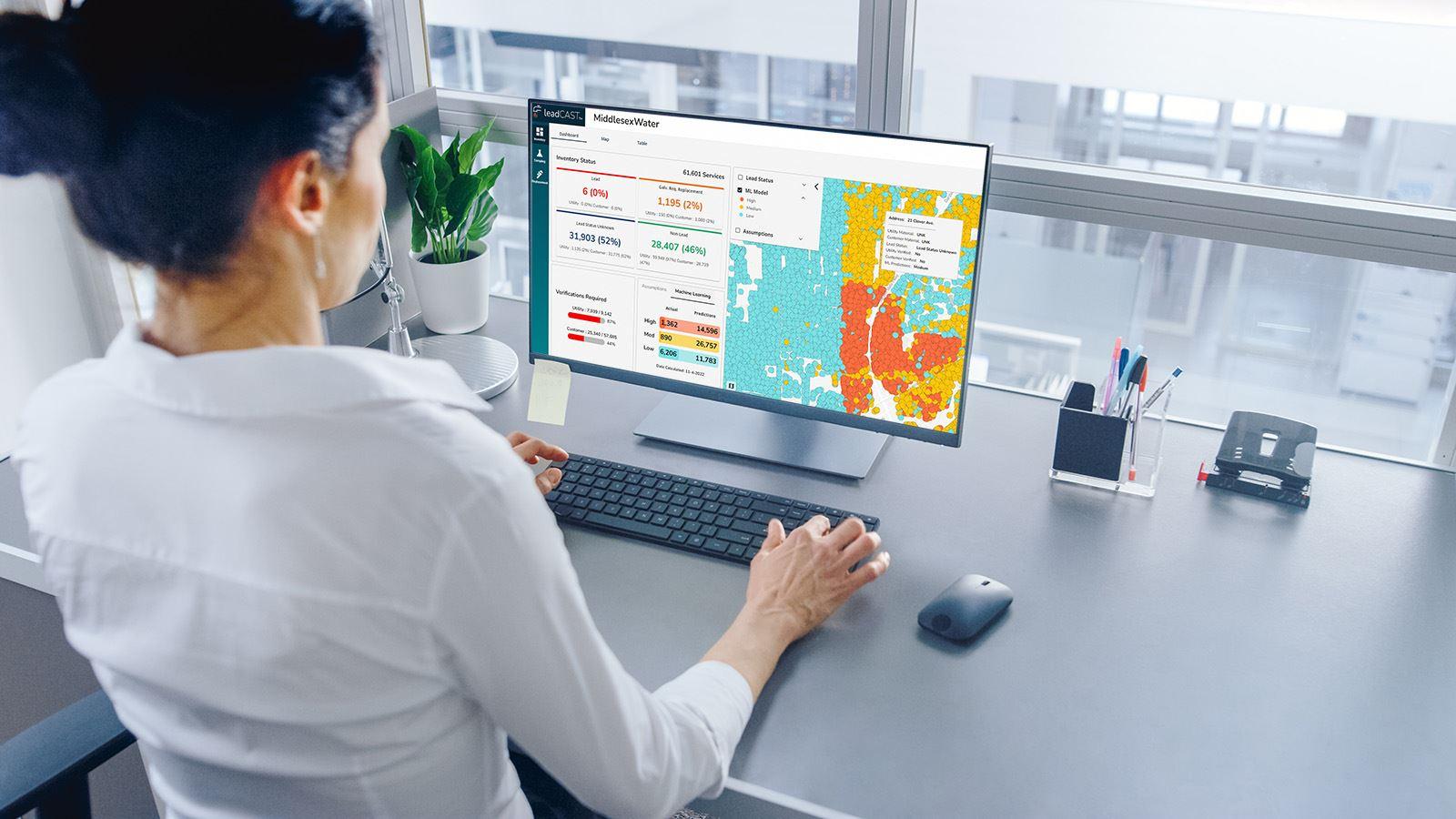
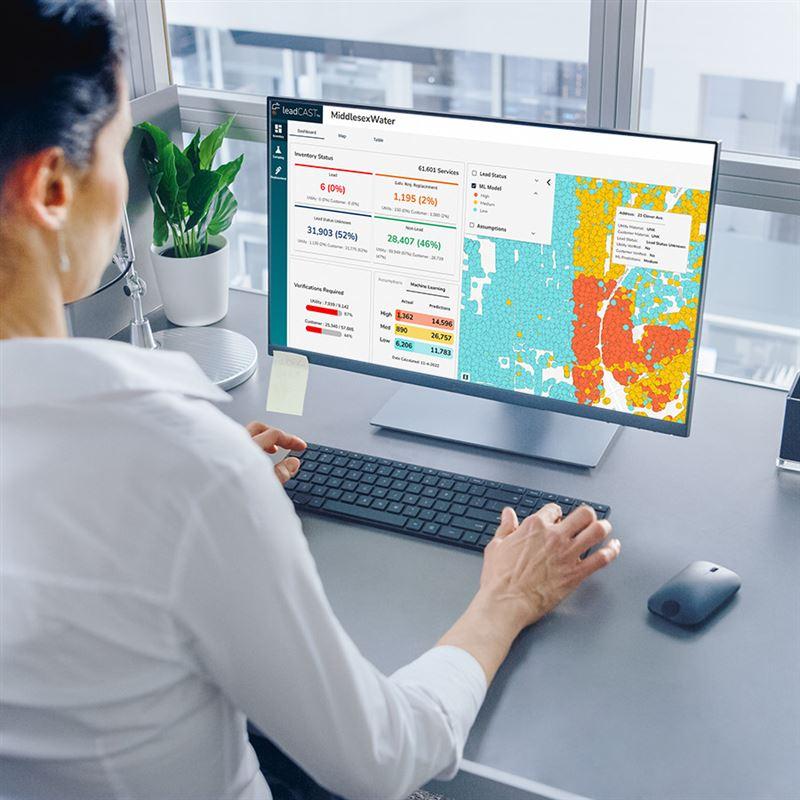